TUTORIAL #3

Luciano Miuccio
University of Catania, Italy
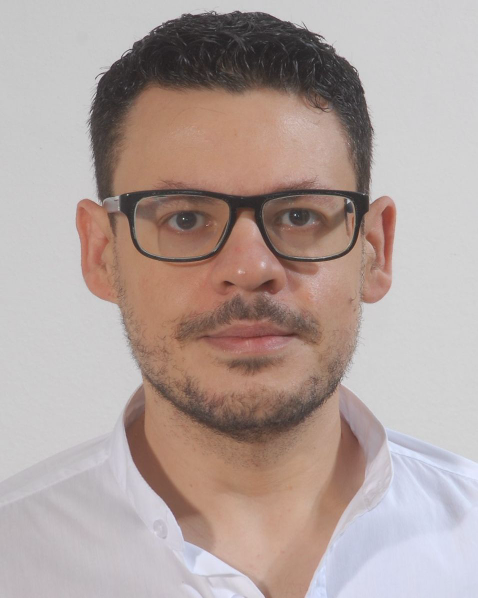
Salvatore Riolo
University of Catania, Italy
The Industrial IoT (IIoT) paradigm is revolutionizing industrial manufacturing by combining advanced Information and Communication Technologies (ICT) with Artificial Intelligence (AI) to optimize industrial processes. Future industries will move away from traditional reliance on wired communication technologies, embracing wireless solutions that allow unprecedented levels of flexibility and adaptability. Specifically, industrial assets (robotic arms, pumps, valves, pistons, etc.) will be equipped with wireless nodes, enabling a multitude of new applications, such as real-time monitoring, autonomous robotics, and predictive maintenance. These applications will have highly heterogeneous characteristics in terms of traffic types, number and type of devices, service area, device distribution, mobility, and so on. In addition to the heterogeneity challenge, several new industrial applications are characterized by extremely stringent Quality of Service (QoS) requirements in terms of end-to-end latency and reliability.
Since existing wireless technologies cannot meet these requirements, various AI-based technological advancements have been proposed in both academia and industry. From Release 16, the 3GPP introduced the Non-Public Network (NPN) paradigm, which enables new AI-based solutions tailored for industrial use cases, paving the way for new research trends oriented toward 6th Generation (6G) networks. In this context, Reinforcement Learning (RL) allows networks to dynamically learn and adapt to the industrial environment and the QoS requirements of industrial applications without constant human supervision or the use of expensive datasets. Within an NPN RL-empowered architecture, two case studies are analyzed.
The first case involves the “mobile control panels” use case, where portable wireless devices, such as tablets or wearables, allow operators to interact with machinery, production systems, robots, or other industrial equipment within the work environment. The portable devices generate packets following different traffic patterns as defined by 5G-ACIA. Through a centralized learning paradigm where the RL agent resides in the Base Station (BS), radio resources are dynamically allocated to the portable devices without prior knowledge of the generated traffic.
The second case involves the “process monitoring” use case, where wireless IoT devices send data to an aggregator node to monitor and analyze industrial processes to ensure proper operation, safety, and efficiency. The goal is to generate a MAC protocol for collision-free radio channel access, tailored to the characteristics of the industrial scenario (traffic model and channel model). The approach involves a distributed learning paradigm based on Multi-Agent Reinforcement Learning (MARL), where learning agents reside in the IoT devices.
In both cases, the proposed solutions are designed to be implementable in a real industrial scenario, eliminating the need for an omniscient environment that provides states and rewards to the learning agents and considering the signaling overhead.
List of the Main Topics:
- Definition of industrial use cases and QoS requirements as per 3GPP and 5G-ACIA.
- 3GPP-defined NPN industrial network architectures.
- Overview of single-agent and multi-agent reinforcement learning frameworks and their importance in sixth-generation wireless networks.
- Case Study 1: RL-based radio resource scheduling for IIoT networks.
- Case Study 2: MARL-based MAC protocol learning for IIoT networks.
Luciano Miuccio received the master’s degree (with honoris) in telecommunications engineering, and the Ph.D. degree in systems, energy, computer and telecommunications engineering (with the additional label of “Doctor Europaeus”) from the University of Catania in 2018, and 2022, respectively. He was a visiting researcher with the Centre for Wireless Communications (CWC), Oulu, Finland, for five months in 2022. He is currently an assistant professor at the Department of Electrical, Electronics and Computer Engineering of the University of Catania. His scientific interests include green networking, NOMA techniques, meta-reinforcement learning for communication, and AI techniques for B5G/6G radio resource management. He presented several papers in prestigious machine learning for communications SAC (IEEE GLOBECOM and IEEE ICC). He served on the technical program committees of various IEEE conferences, including IEEE ICMLCN, and chaired a session on SAC machine learning for the IEEE ICC 2023 conference.
Salvatore Riolo received the master’s degree (with honoris) in telecommunications engineering, and the Ph.D. degree in systems, energy, computer and telecommunications engineering from the University of Catania, Italy, in 2017 and 2021, respectively. He was a visiting researcher with the Centre for Wireless Communications (CWC), Oulu, Finland, for six months in 2022. He has been an assistant professor of telecommunications with the Department of Electrical, Electronics and Computer Engineering (DIEEI), University of Catania, since January 2022. His scientific interests include radio resource management for B5G/6G networks, and the application of supervised learning and reinforcement learning to radio access networks. He presented several papers at prestigious conferences on the topic of ML for network management, and reinforcement learning techniques for communications. He served on the technical program committees of various IEEE conferences.
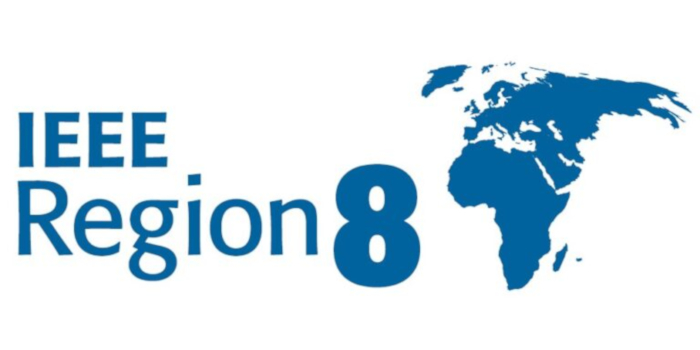
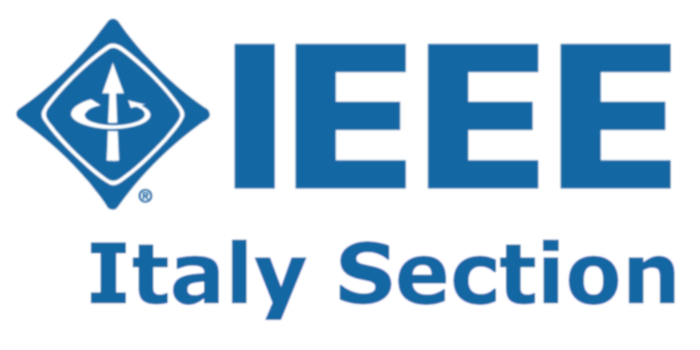
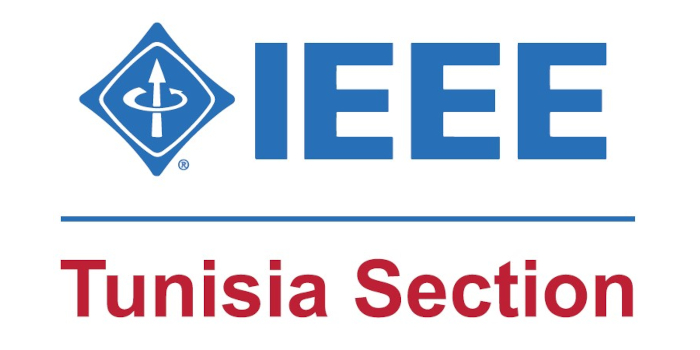
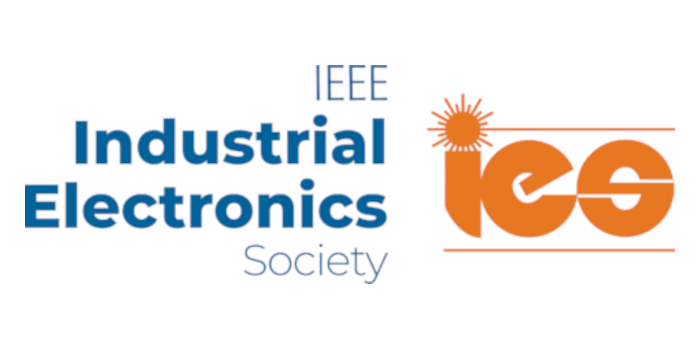
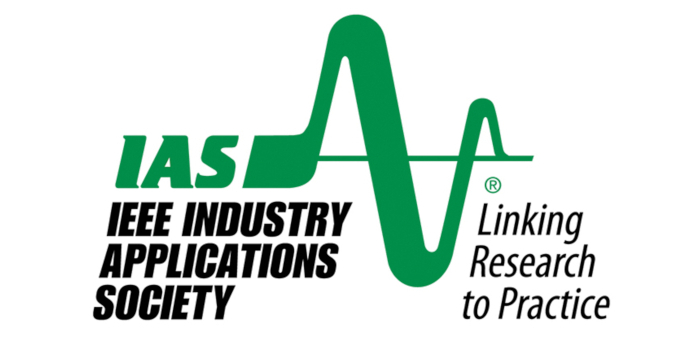
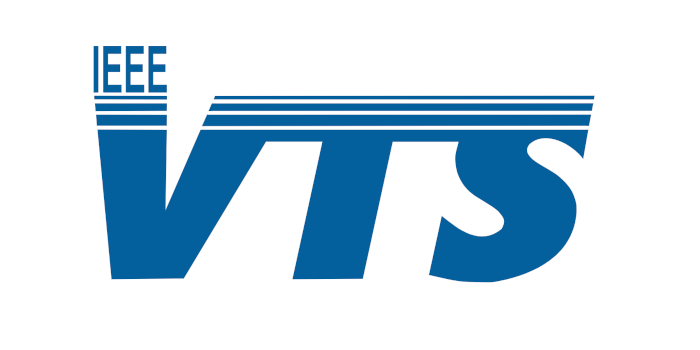
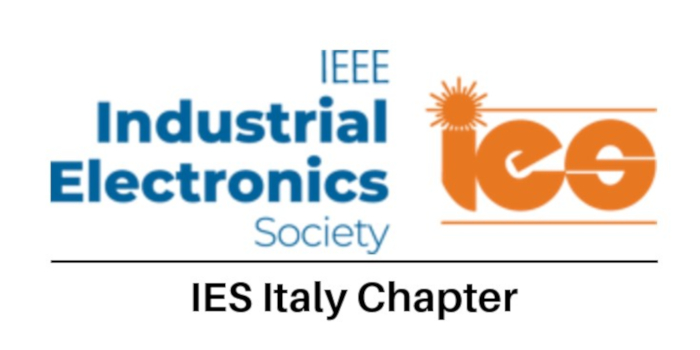
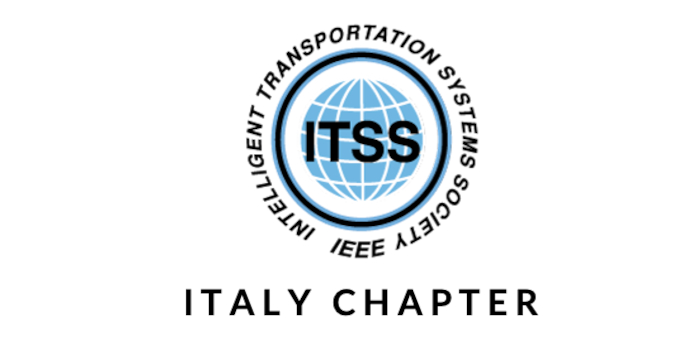
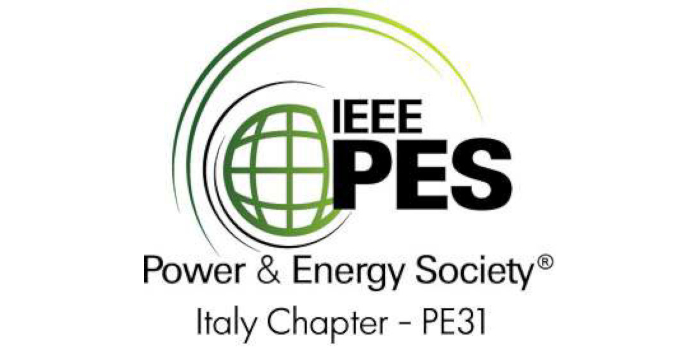
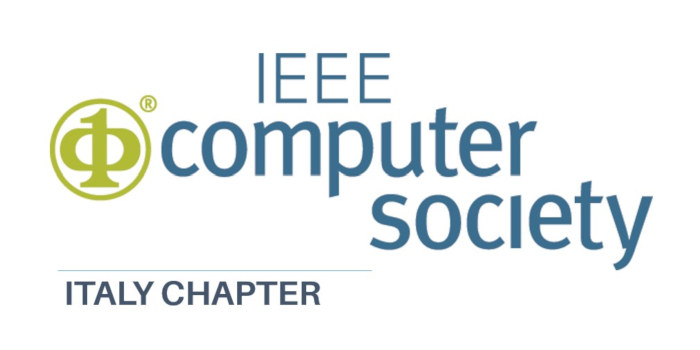
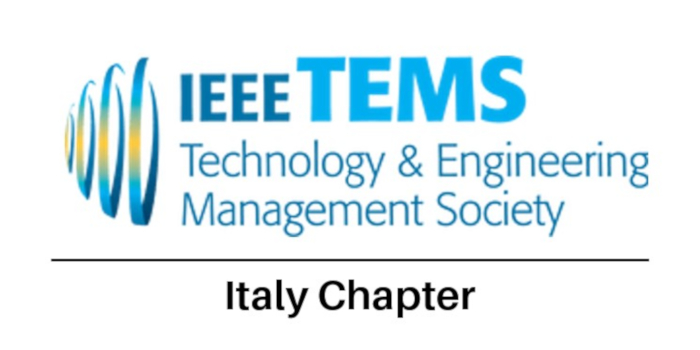

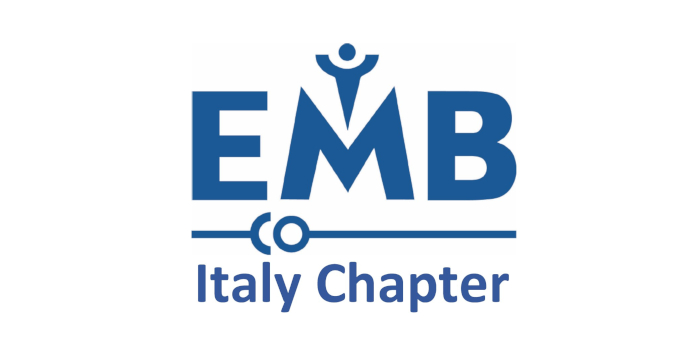
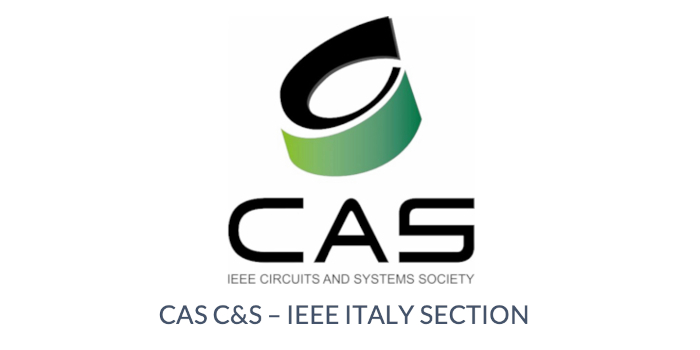
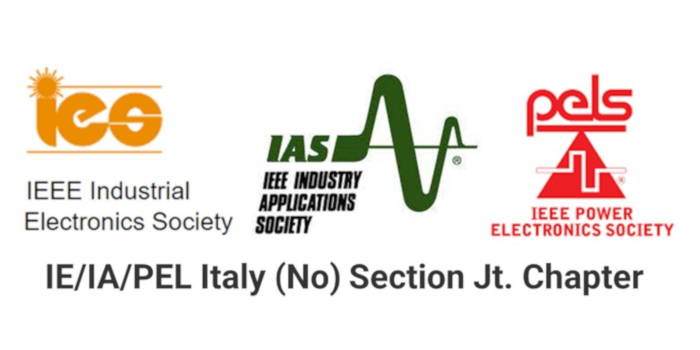
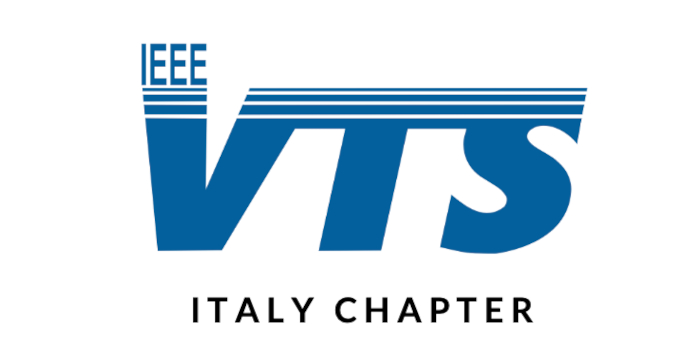
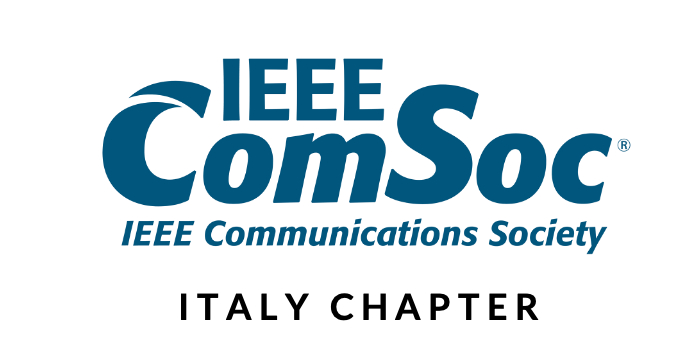
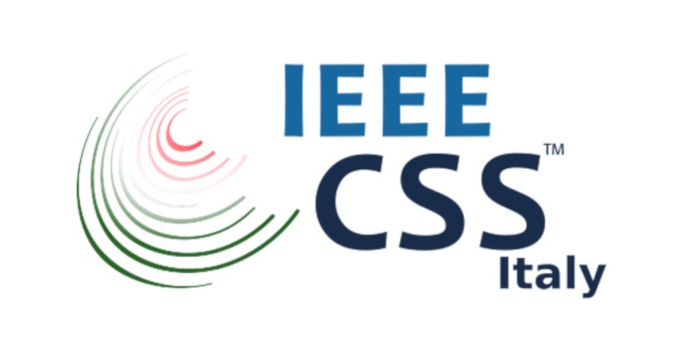
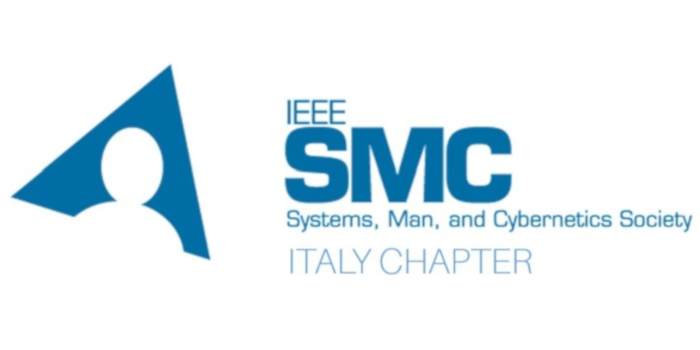

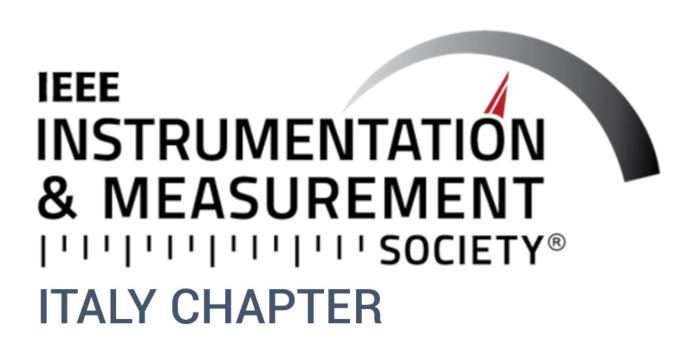
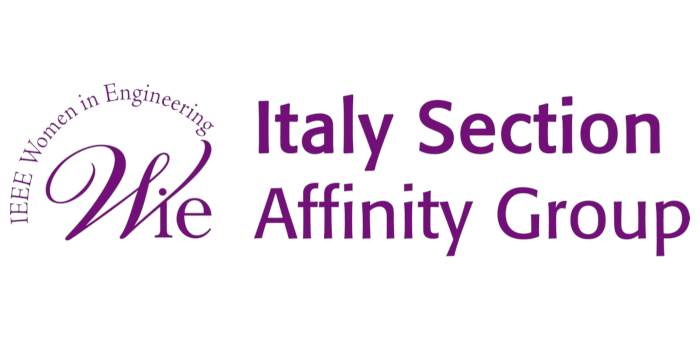
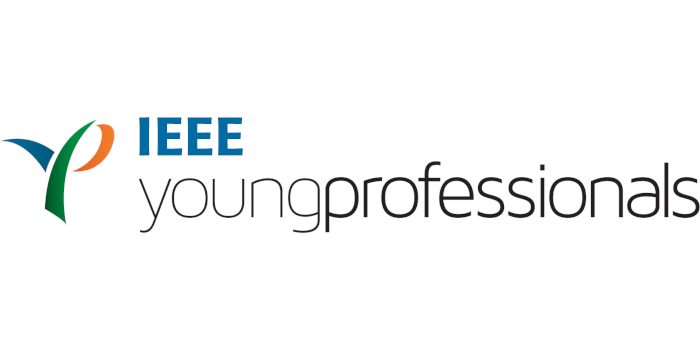
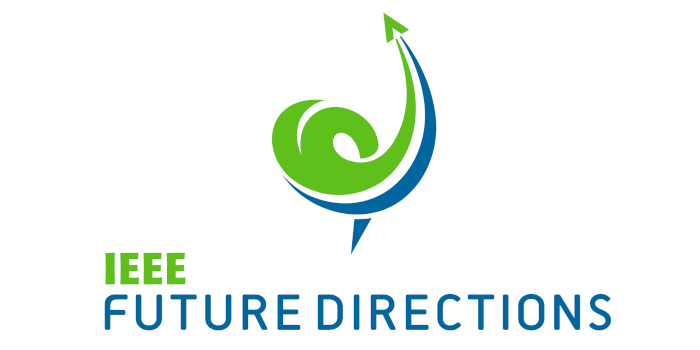